Industry Keynotes
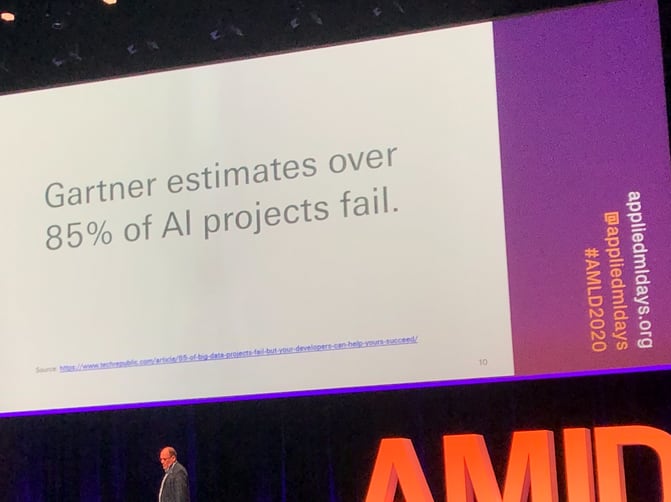
The industry keynotes were mostly short (15min) highly polished presentations giving a quick glance on how various parts of the industry are applying machine learning in their domain:
- Roche was showing how data is driving patients health - something that we will definitely see in the future. While I think it seems like a good thing, I think we don't fully understand how drastic this shift will be.
- Bühler (mostly known for their big agricultural food processing technology) was showing how to use deep learning computer vision to determine the quality or grains or how to use dynamic time warping to detect machine anomalies.
- SBB gave a quick glance on how they use computer vision to constantly control the quality of their tracks, and
- Novatis and Microsoft argued why marrying the pharma and tech industry is a good idea: Novatis has an abundance of processable data and Microsoft apparently the computing power and the skills to process it all. I am excited what how this partnership will turn out.
Seeing these tracks made me realise that big tech giants have indeed successfully moved AI tech into established industries. While all of these endeavours seemed very impressive there were also talks about the fact that 85% of all AI projects fail in a corporate setting. So I guess under the hood this shows that there is still a lot of experimentation going on.
Evening Keynotes
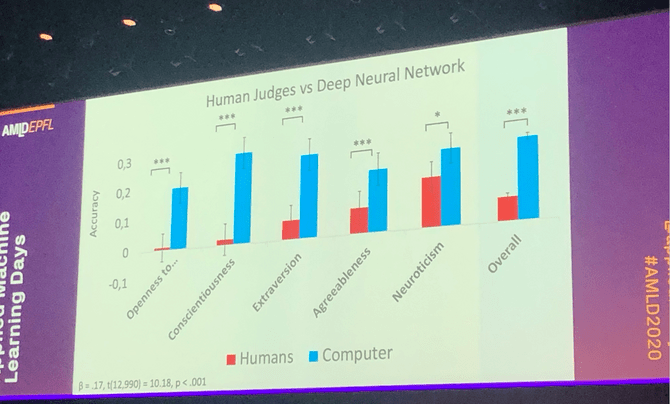
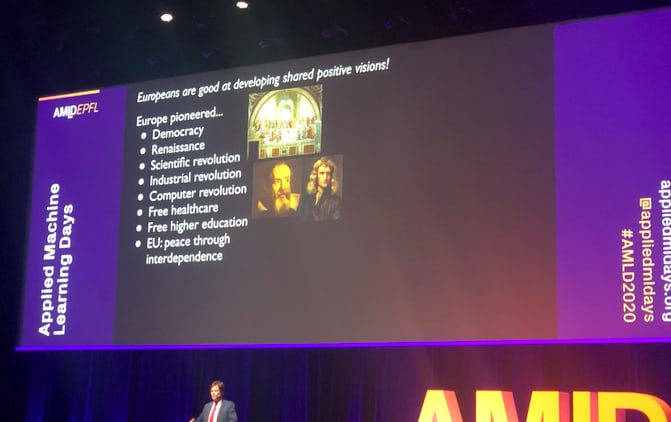
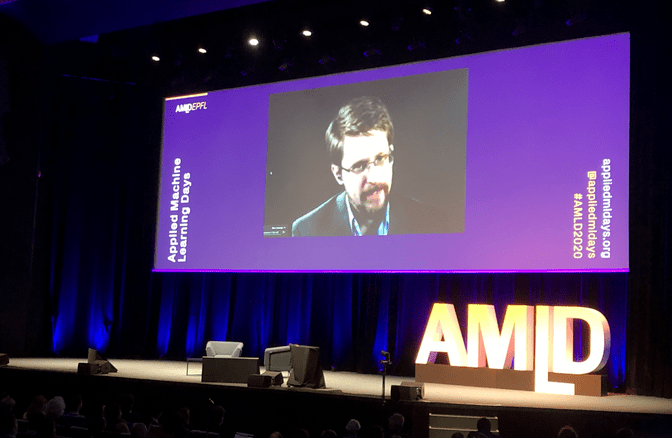
The evening was reserved for three very special long keynotes, all of them focused on the role of AI in our society - something that has somewhat been a very prevalent topic this year:
- The evening started with a great presentation of Michal Kosinski (the researcher that unveiled the dark tactics of Cambridge Analytica) who gave a talk on the end of privacy. A title that seems dystopian at best, yet spot on, since he was showing how deep learning based on face detection outperform humans at predicting their personality traits. So we have definitely arrived at a time where an algorithm is definitely better at predicting if a person is neurotic, or introverted than a person. I really thought that this is something that's reserved for us humans, but apparently it is not. While the use of face detection in public is heavily discussed, it will be probably impossible to close this Pandora's box. If you are interested in this topic, we have actually written a blog post with code on how to predict your personality traits from your facebook likes.
- Prof Tegmark from MIT had a more positive view on the future role of AI in our society: His talk was basically a quick glance over his new book Life 3.0 arguing that AI can greatly help society to improve on the development of sustainable goals. He also argued that researchers should come together and take a stance on the use of AI weapon systems, similarly like researchers did back in the days on Biological weapons. Europe can take a central role here, since historically we have been quite good at creating shared visions for the future.
- Finally the big show was reserved for Edward Snowden, who attended via a video link from Moscow. His talk was mostly a mix of his former positions on privacy and government supervision, most of which he also covers in his current book permanent record. I took away an interesting anecdote from the current implications of the GDPR law and facebook, where a researcher tried to make Facebook give him really all the data they have on him (so all of the metadata, behavioral data, etc..). While the self service website gives you a possibility to get the obvious data, Facebook declined to give him the machine readable metadata with the argument that its not easily consumable by a layperson - something that seemed like a loophole in the current GDPR regulation.
Poster Sessions & Smaller Talks
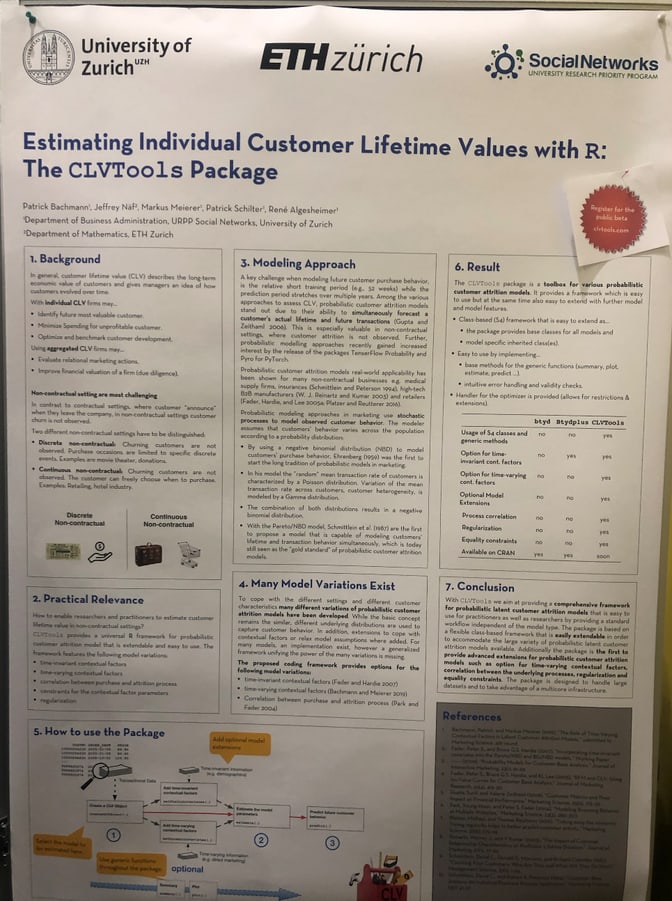
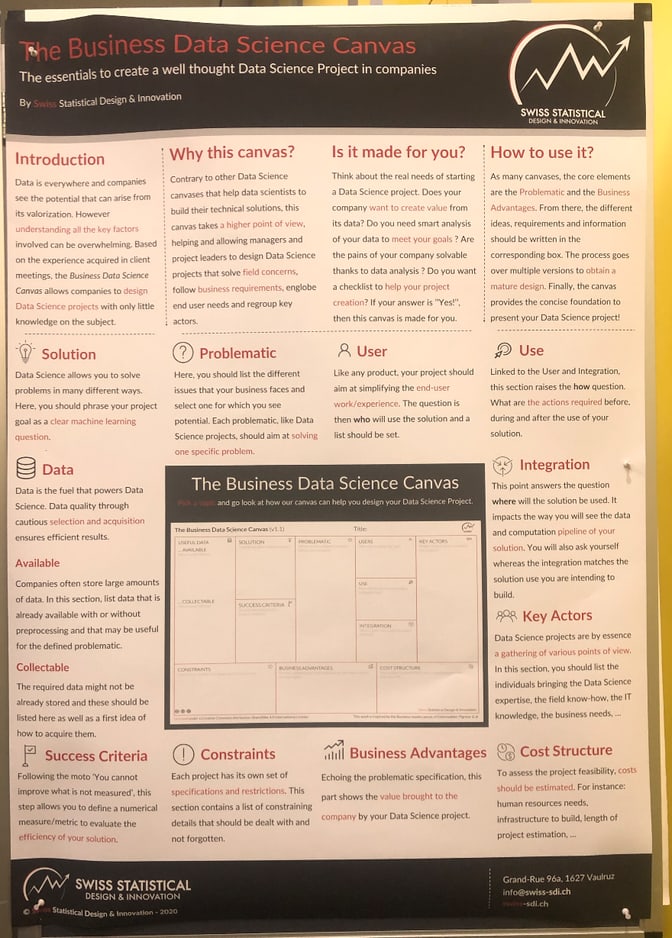
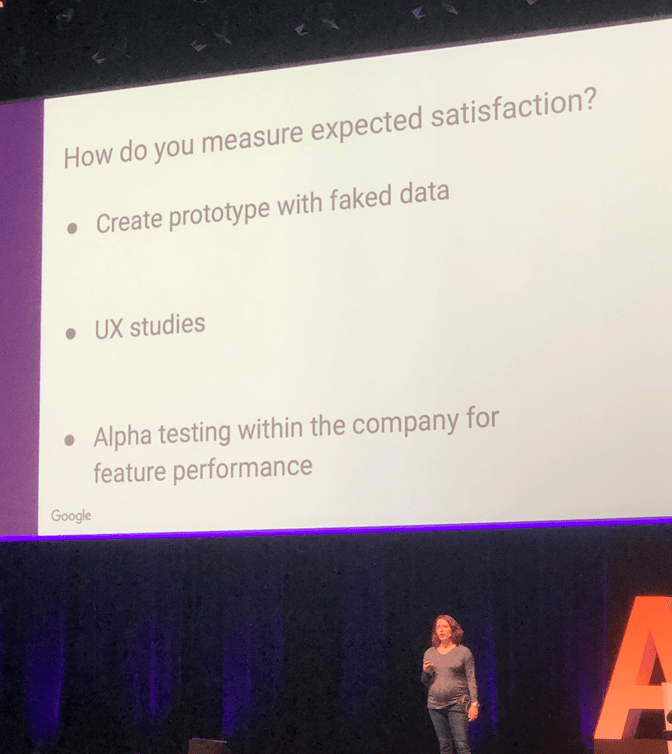
Here are three things I found very applicable and interesting in the poster sessions:
- WildNLP: An open source framework for making sure your NLP models work in the wild - it helps you to corrupt your text on purpose to test how stable your NLP model really is.
- CLV Tools Package: A new R package to estimate customer lifetime value of customers, including the possibility to see what would happen if we do an intervention. Same idea like lifetimes for python. Predicting the CLV of a customer definitely is a strong context that seems applicable in almost every industry.
- The Business Data science Canvas: An adaptation of the lean business model canvas to the data domain. While it seems a bit of a stretch, it's definitely something that our Bizdev Emilie would love. We also have developed a smaller version of it that we use regularly to kickoff projects with our customers.
- Megan, from the Google team in Zürich, showed very interesting insights on UX and ML: While generic ML metrics tend to be OK for comparing ML models amongst each other they will not tell you if your model sucks when you put it in front of users. This is something weāve been seeing with our customers too - that's why in our team we try to evaluate in a lean way as fast as possible if the final product is considered useful.
Tracks
The tracks were really the core of the conference. There were more than 20 different tracks on various topics and I tried my best to soak up as much as I could. Here are some key takeaways from the ones that I attended:
- AI and and leadership: I learned how much apparently the IEEE organisation is pushing standards of an ethical use of AI. I will look forward to reading their new paper they are launching in February on āA call to action for businesses using AIā, which is going to provide more orientation sketching an AI ethics readiness framework. On the macro scale I liked their simple formula of People+Planet+Profit as a positive example of setting new balanced goals.
- AI and Education: I just attended one talk from Squirrel AI learning which seems to be massive in Asia. While at Liip we are trying hard to figure out how to blend AI and learning well I felt that either this company has it all figured out or that this company is somewhat flueling inflated expectations for this domain. At least the "learning path" (see video) to me seemed a bit chaotic, but who knows :)
- AI and edge: Definitely a field with a lot of momentum as we are trying to make the internet of things smart while maintaining privacy. Something that I will definitely look into is federated learning a simple idea where the data is kept at the client while the model weights are exchanged. We will see if this is a great way to protect user privacy in machine learning on the edge.
- AI and cities: A very interesting panel where I noticed that we are very fast moving from simulated models of cities, to models where we have a lot of actual data. There were companies like POSMO, which created a platform for collecting user movement data on yourself while making it also possible to share it with researchers. FAIRTIQ showed how they use ML to predict the users mode of transportation using his mobile phone sensor data. My favorite anecdote: When you traveled with a funicular in Fribourg during the beta phase, you were travelling for free, since the model assumed you were walking because it is so slow. A fascinating learning for me were meta models on simulation. The idea is simple: Instead of simulating a complicated city traffic system researchers used it to generate data and then train a model on this data. Now instead of running a simulation for 30min they simply use this meta-model to predict the outcome given some parameters e.g. waiting on red traffic lights in just seconds. Another interesting idea was to build digital twins of buildings where we collect all of the sensor data of a city and then rebuild it digitally live in an AR environment. In a nutshell: a lot of interesting things seem to be going on in this area.
- AI and NLP: It's not surprising that this track attracted a lot of visitors. Some things that I took away from it: Researchers are finally tackling the problem of making NLP work in multiple languages. For wordnet we now have BabelNet and Verb Atlas. There is also SyntagNet, which allows us to go from a word vector space model to a sense vector space model. Interesting advances in making chatbots were shown by researchers who developed PLACAT: Basically a system that allows us to distinguishes if the user want to chat or really find out some truth. Depending on this we forward him to different parts of the chatbot. Companies like doodle showed entertaining ways on how they use NLP models to fight spam or Bloomberg showed how they try to automatically augment news to extract relevant structured information. Two companies showed me how close useful and creepy solution can be: A very useful solution to me seemed to be the development of an AI Android keyboard. By monitoring what kids type into their mobile chat it offers a way to detect hate speech or critical/suicidal conditions and allows parents to intervene. A totally creepy solution seemed the development of Deeptone: A solution analyzing speech patterns to figure out if users are happy and energetic. Apparently it is used in call centers to āhelpā their employees to āimproveā their client work. This definitely crosses the line for me.
Summary
The venue was big and this year there were a lot of people who attended. A machine learning conference with almost 2000 participants that goes on for more than 3 days definitely shows that there is a big buzz about this topic. This year I found even more big swiss companies (e.g. Swisscom, Novartis, Roche, Bühler, AXA, etc..) which were heavily recruiting new young academics from university to staff the need for data analysts, data scientists or data engineers.
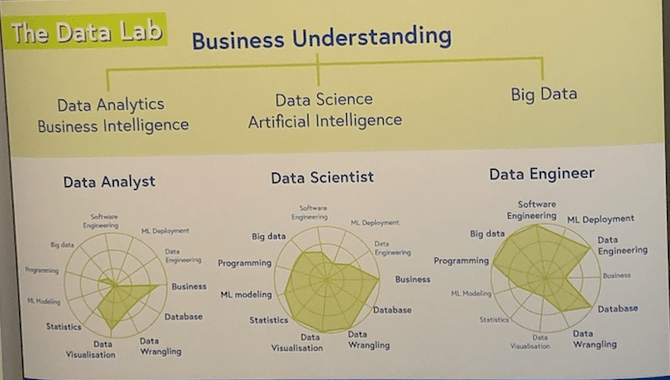
On the other hand these academics were indeed the big majority of the participants and gave the majority of the talks. So this dsicrepancy lead to an interesting āknowledge gapā: I noticed that I either attended tracks where academics were presenting their research papers or - on the opposite of the spectrum - 50ish year old CTOs/CDOs/CEOs in fancy suits were roaming the big center stage and giving very high level talks on: How to be a truly data driven company.
While both sides draw a different crowd and both have their benefits, what I lacked a bit was the applied part: e.g. people showing what code they wrote, what worked / what didnāt and sharing their hands on lessons/best practices they learned the hard way from their projects. Maybe such insights are best served on (shameless advertising: join us in our monthly meetup in Zürich in our arena) - or maybe we as data scientists/researchers/engineers all just need to find a way to be more open about our work and methods while maintaining the intellectual property of our employers.
Democratization of AI
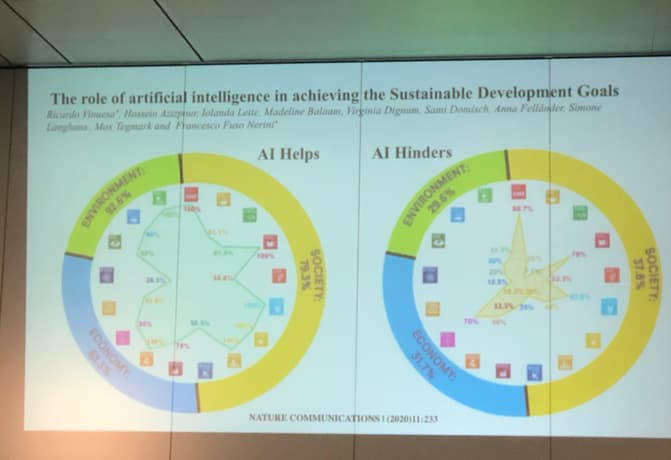
While I obviously took away some interesting new concepts, new interesting open source projects and new approaches in different domains, I also took away my own high level learning from this conference. It came in form of a question that seemed prevalent at the conference:
How can we create an AI that truly serves the people?
As we have seen, taking a stand on the ethical and privacy concerns seems to be something the community is currently thinking about. Yet for me the question above translates into another question that I think we should solve next:
How can we create AI agents that truely act on your behalf?
And obviously such personal AI assistants will have to be created in such a way that they act in your best interest. Since no laymen will be able to tell if the AI is acting in his best interest we can only hope that making such systems open source might somewhat help to spark a discussion what it acting in your best interest really is. I think that this direction is radically different on how we are using AI today. Right now it is only reserved for big companies with big budgets, big computing power and enough data and skills.
Basically thinking about personal AI-assistants means democratizing AI and making it available for everyone. Making it transparent. Making the data that these systems need accessible, while maintaining the users privacy. Federated learning and open source seem to be two very plausible approaches to this problem. I am also sure that open data can make a big contribution towards such agents, but we might need to rethink what open data means in the age of AI.